New frontiers of human-machine interaction: artificial intelligence (AI) and the arrival of smart assistants
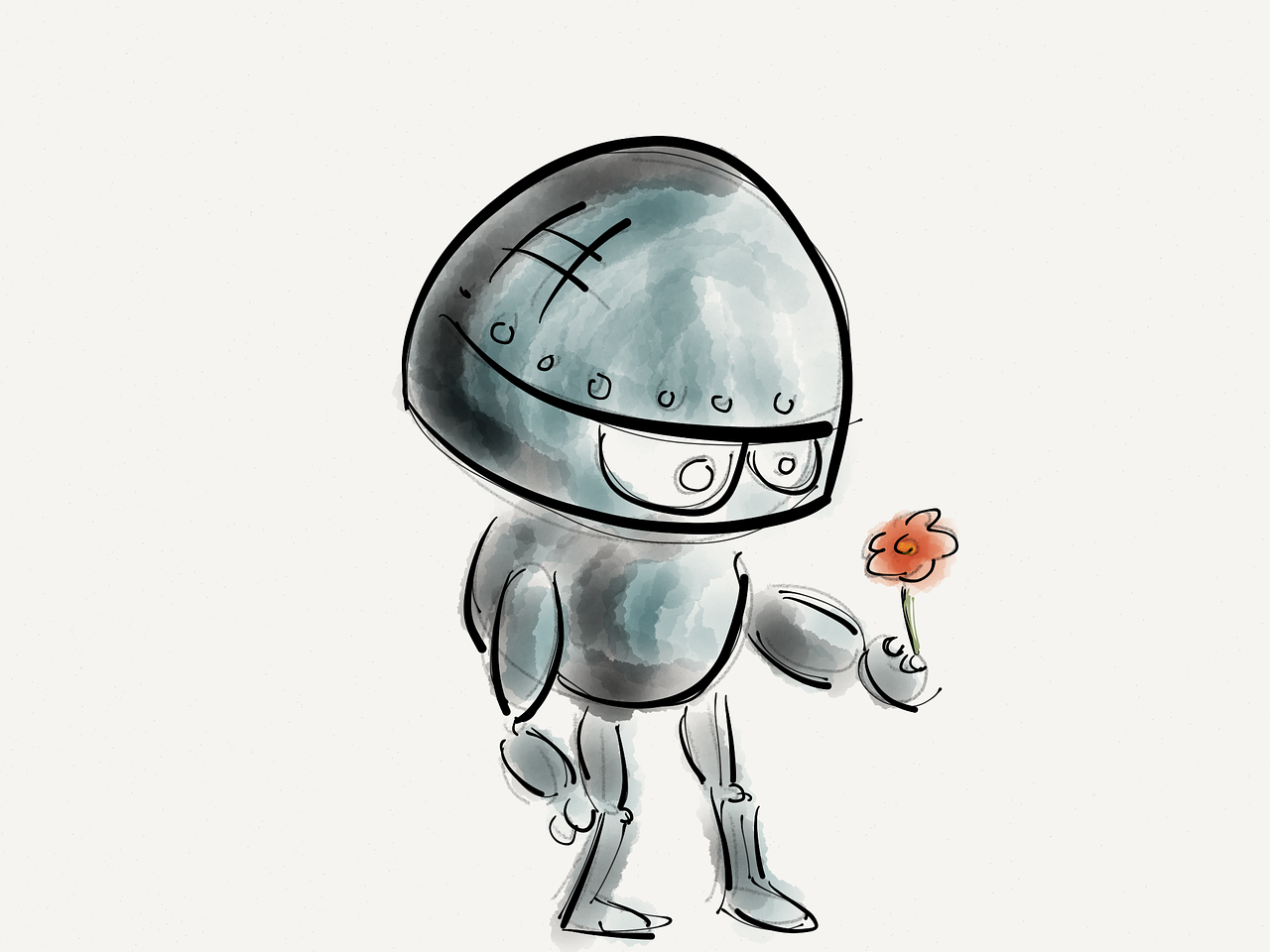
We live parallel lives. One in the natural environment, where the sustainability of our behaviour is a fundamental condition for biodiversity. The other in a digital ecosystem where our behaviour is based on sequences of clicks. To tackle the complexity and challenges that we meet, we increasingly rely on electronic devices and the digital river overflows into the natural world over which we no longer act directly, but through a computer mediated experience. The user interface is the new territory of the mind, a non-place that becomes a primary tool through which we learn and act, in work, fun and social relations.
Creating user interfaces, which make the use of systems, digital products, environments and services as intuitive and simple as possible for users was one of the objectives of interaction design, in which user-related issues guide the design process more than technical issues.
The involvement of users in the design step must take into consideration the fact that every person has their own perception of reality. These differences are the result of different life experiences, the reprocessing performed by the brain of images sent by the retina and selective attention to what is around us, moving our focus onto certain elements rather than others. In fact, Heraclitus stated that men are often deceived in their knowledge of things that are manifest and reason does not always succeed in exercising effective control over the wide range of illusions. Therefore, perceived reality has an extremely subjective component.
It is precisely towards perceived reality that technology has been taking steps for many years, extending and/or completely overlapping with real reality. In 1957 Sensorama was invented, the first example of virtual reality, by Morton Leonard Heilig. Sensorama consisted of a cruiser in which users sat and watched three dimensional videos accompanied by smells, air and sounds.
Virtual reality became more common because of the evolution of mobile devices which, with the same calculation power as computers and high definition displays, became a more than valid tool for the gaming and entertainment world.
Hence, products like Cardboard by Google and Gear VR by Samsung were created, essentially boxes with a housing for the device.
These results were obtained first by involving the main human senses, i.e. sight and hearing, eventually involving all the others.
The study of Artificial Intelligence has moved towards the creation of systems that can reproduce the characteristics of the human brain such as perception, reasoning, intuition and understanding, in order to reproduce increasingly “human” tasks, for making human-machine interaction as close as possible to human-human interaction, it being understood that awareness, affection and emotion still remain a prerogative of human beings only.
A great step forwards came about with the use of neural networks, networks made up of artificial neurons that work in parallel and are based on statistical data. To understand what a neural network is, it is helpful to understand how Perceptron, the first artificial neuron created between 1950 and 1960, works. A perceptron has different binary signals as inputs and produces only one output.
Each input signal is associated with a weight w that allows added value to be given to the various input data. The output comes from a simple function.
By changing the weights and the threshold value, different decision-making models are obtained. What is wanted from these modules is that a small change to the weight of the input causes a small change to the output. However, this characteristic cannot be obtained with perceptrons therefore sigmoid neurons must be used. These neurons, like perceptrons, have different inputs, each of which can take on a value comprised between 0 and 1. Each input is associated with a weight and has a threshold value. The substantial difference with perceptrons is that the values returned by sigmoids are comprised between 0 and 1.
A distinctive element of neural networks is machine learning, which can be defined simply as follows:
“a program learns if there is an improvement in performance after a task performed”.
Arthur Samuel, one of the pioneers of AI, defined machine learning as the field of study that gives computers the ability to learn without being explicitly programmed.
What happens is that whenever a system based on neural networks is used, the system “learns” and improves, balancing the weights so as to minimize error.
Let’s suppose that the information x0 is processed. If the signal is strong enough the neuron passes into the active state, i.e. the weight of the w0x0 connection is such that the neuron processes the input data and produces the related output through the activation function.
By activating some neurons and not others and reinforcing the connections between the neurons, balancing the weights so as to minimize error, the system learns what is important and what is not.
An example of neural networks comes from Google Neural Machine Translation (GNMT), the system developed by Google and introduced in November 2016, to improve the precision and speed of Google Translate, a known instant translation and reading service.